The Ultimate Guide to AI Cost Analysis
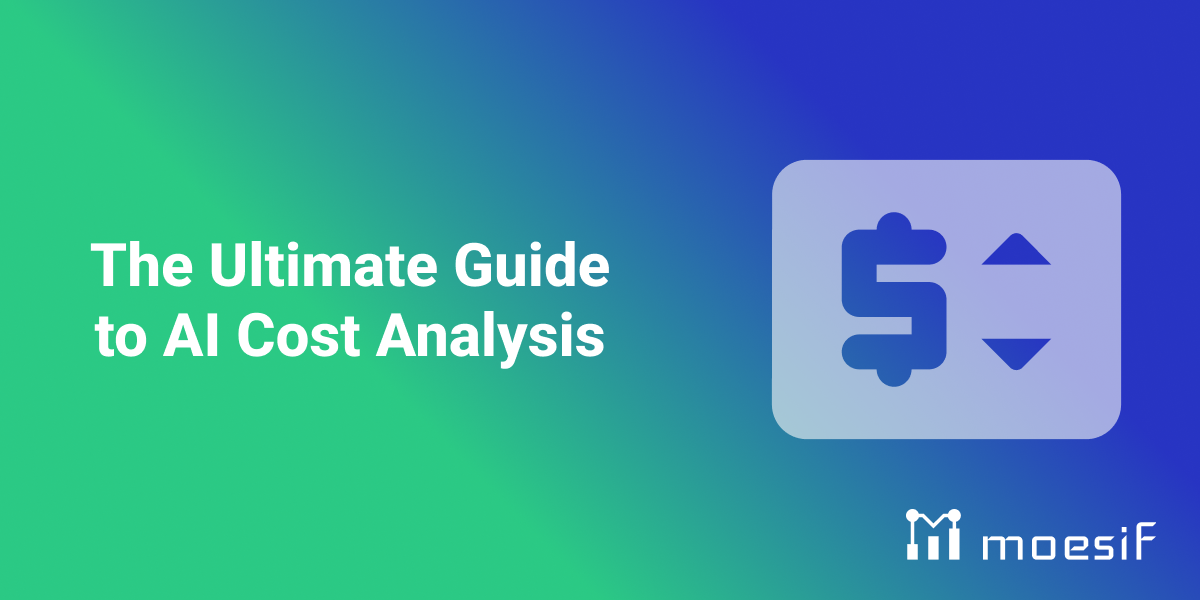
Artificial intelligence (AI) has quickly become integral to businesses across industries. Whether utilizing Large Language Models or other Machine Learning platforms, AI-powered tools offer immense potential for growth and efficiency. However, as AI adoption surges, so do the associated costs of building and supporting AI apps. For companies that use AI as part of their product offering, understanding and managing the costs associated with AI capabilities is crucial to ensuring AI initiatives deliver a sustainable return on investment.
This comprehensive guide will explore AI cost analysis from many angles. We’ll explore what it entails and why it’s important and provide you with a step-by-step approach to calculating and optimizing your AI expenses. We’ll also discuss how leveraging tools like Moesif can streamline the process and accuracy of AI cost analysis, giving you a clear view of your AI spending. Let’s start by looking at what AI cost analysis is and why it’s essential to keep track of.
What is AI Cost Analysis?
AI cost analysis evaluates and understands the financial implications of developing, deploying, and maintaining AI systems. It involves breaking down the various cost components associated with AI, from initial research and development to ongoing operational expenses. Much of the time, the main reason for tracking this is to ensure that profit margins from an AI platform are in line with expectations and, more importantly, to ensure that the cost of your AI offering does not exceed the revenue being generated.
A comprehensive AI cost analysis typically includes the following elements:
-
Infrastructure Costs: This encompasses the cost of hardware (servers, GPUs, etc.), cloud computing resources, and storage needed to train and run AI models.
-
Data Costs: Acquiring, preparing, and labeling data for AI models can be a significant expense, especially for complex projects requiring large datasets.
-
Development Costs: This covers the salaries of AI engineers, data scientists, and other specialists involved in building and refining AI models. It also includes the cost of software tools and licenses.
-
Operational Costs: These are ongoing expenses associated with running and maintaining AI systems, such as energy consumption, monitoring, and updates.
-
Unexpected Costs: AI projects can encounter unforeseen challenges, such as model retraining or debugging, which can add to the overall cost.
AI cost analysis gives organizations the insight they require to make informed decisions about their AI investments. The outcome of this type of analysis helps them ensure that AI projects are technically successful and financially sustainable.
Why AI Cost Analysis is Important
Understanding the financial implications of your AI initiatives, or any technology initiative, is essential. As much as we would like to think that the goal of our latest AI project is to change the world, we can’t get things to scale if they’re not making the money they need to sustain themselves and drive further innovation. Compared to prior generations of technology, AI is extremely expensive to deploy and manage, requiring massive amounts of computing power and data. This underscores the importance of making AI cost analysis a regular part of reviews for the business and technical teams involved. Here are a few reasons why AI cost analysis is important:
1. Ensuring Profitability:
AI can be a powerful tool for driving revenue and reducing costs. However, the initial investment and ongoing operational expenses can quickly escalate. By analyzing costs, you can identify areas of inefficiency, optimize resource allocation, and ensure that your AI projects generate a positive return on investment.
2. Resource Optimization:
AI projects often involve significant resources, including computing power, data storage, and specialized personnel. Cost analysis allows you to track resource utilization, identify bottlenecks, and optimize resource allocation to maximize efficiency and minimize waste.
3. Risk Mitigation:
AI projects are not immune to risks like unexpected technical challenges, model drift, or data quality issues. These risks can lead to cost overruns and project delays. Cost analysis helps you anticipate potential risks, allocate contingency budgets, and take proactive measures to mitigate their impact.
4. Data-Driven Decision Making:
Cost analysis provides valuable data insights that can inform strategic decision-making. By understanding the cost-benefit tradeoffs of different AI approaches, you can make informed choices about which projects to prioritize, which technologies to adopt, and how to scale your AI initiatives.
5. Transparency and Accountability:
AI projects often involve multiple stakeholders, including executives, engineers, and financial teams. Conducting a transparent and comprehensive cost analysis can ensure that all stakeholders clearly understand the financial cost and impact of AI initiatives, fostering accountability and trust.
AI cost analysis goes beyond saving money. These analyses help everyone involved make informed decisions, maximize the value of your AI investments, and ensure an AI initiative’s long-term success and sustainability.
How to Do an AI Cost Analysis
Now that we understand the fundamentals of AI cost analysis let’s examine the exact steps to uncovering AI costs. Below, we will cover three steps required to calculate your AI costs and then an additional step to outline ways to improve them.
1. Identify Cost Components
In this first step, you’ll need to identify various costs associated with building and supporting your AI product. The areas you’ll want to focus on are:
-
Infrastructure: Estimate the cloud computing costs for running the API infrastructure (servers, load balancers, databases, etc.). Consider the volume of API requests across all clients, the average request processing time, and the required computing resources per request.
-
Model Inference Costs: If you’re using cloud-based AI models (e.g., GPT-3), calculate the costs associated with each API call based on the number of input and output tokens and any additional charges for context size or model complexity.
-
Data Costs: Factor in any ongoing costs for model training or fine-tuning, especially if you’re offering customization options to your clients.
-
Development: Calculate the salaries (or hourly rates) of your AI engineers and developers responsible for building and maintaining the API, including ongoing feature development, bug fixes, and performance optimizations.
-
Operational: Include the ongoing costs of running the SaaS API platform, such as server maintenance, software licenses, customer support, monitoring tools, and API documentation updates.
-
Client-Specific Costs:
-
API Usage: Track the number of API requests made by each client, as this will directly correlate with your infrastructure and model inference costs.
-
Support: Consider the cost of providing technical support to clients for API integration and usage issues.
-
Customization: If you offer customized models or features through the API, track the costs associated with each request.
-
Some of these aspects may be hard to quantify, however, getting somewhere in the ballpark or an estimate is generally sufficient. This is especially true if you’re trying to do some predictive cost analysis.
2. Quantify Costs and Usage
Next, if we are looking at a historical period, we will need to figure out the actual usage of our AI product for the period we are analyzing. If you are trying to forecast usage, use estimates for these factors. Since our example is focused on an AI API, we can leverage API analytics tools like Moesif to gather detailed usage data for each client. Metrics to focus on include:
-
Number of API requests
-
Input/output tokens per request
-
Context size (if applicable)
-
Error rates
-
Latency
Once you have actual or estimated usage metrics, use the data to allocate infrastructure, model inference, and other relevant costs to each client based on their API usage.
3. Calculate Cost per Token/API Call/User
With a clear breakdown of your costs, you can begin digging deeper into the specifics. Although you could look at attributing costs from various angles, popular ways to do so are by token, API call, or user.
In the case of calculating cost per token, one of the most common ways to calculate costs for AI platforms is to aggregate all of your costs and divide them across the total amount of tokens consumed. This will give you an average cost per token, allowing you to dial in your pricing and anticipated margins more easily. When it comes to AI cost, this is the most granular unit that makes sense when calculating the cost of AI services.
Cost per API call, a less common way to calculate costs for AI APIs can be done similarly to tokens. In this case, you’d add up the costs for your API and then the number of API calls made in the same period. From here, you would divide the costs by the amount of API calls, resulting in your cost per API call. Of course, this will give you a less granular look than assessing costs by tokens used, but this can be useful if it makes sense to price your AI API by API call. An example of this, based on some popular examples, might be a content creation API that creates social or blog posts. In this case, you may charge $2 per social post the platform creates, and that functionality is exposed through a single API. If you calculate that the average cost per API call for that endpoint is $0.25, then you can calculate your average profit as $1.75. So, although a bit less common than per-token pricing, for specific applications, especially those targeted at less technical users, pricing and analyzing costs this way may make more sense.
Lastly, you could also take a look at costs per user. For some platforms, users may pay by the user/seat. To calculate the cost of AI in this case, you’d want to once again add up all of your costs for a certain period of time and find the total number of users on the platform. Then, you’ll divide your total costs by the number of users to get your average cost per user. An excellent example of this might be a platform that has added AI capabilities, such as Notion. In the case of Notion, AI capabilities are available to users but are covered by charging per AI-enabled seat via their Notion AI add-on. To calculate the cost per user/seat, once again, you’ll take your total costs to build and support the AI capabilities and divide it by the total number of subscribed users. This will land you at the average AI cost per user. For example, let’s say that the price charged per seat is $8 per month and, on average, the AI cost is $2 per user per month; then we can quickly determine that the profit is around $6 per month for each user that is subscribed.
These are the most common ways to calculate the cost and profit of AI platforms. When it comes to increasing profit, organizations usually have two options: increase prices or decrease costs. Let’s move on to how to analyze and optimize cost and profit.
4. Analyze and Optimize
Once you have metrics around AI cost and profits, you can consider optimizing using some of the following methods.
-
Usage-Based Pricing: Design API pricing plans based on usage tiers, considering the cost per API call or other relevant metrics.
-
Rate Limiting: Implement rate limiting to prevent excessive usage by individual clients, protect your infrastructure, and ensure fair access for all users.
-
Performance Optimization: Continuously monitor API performance and optimize your infrastructure and models to reduce latency and improve response times, enhancing the user experience.
-
Client Profitability: Analyze each client’s revenue generated compared to their API usage costs to assess their profitability. This can guide your sales and marketing efforts, pricing strategies, and customer support prioritization.
By meticulously tracking and analyzing costs associated with your API-based AI chatbot service, you can gain valuable insights into your business operations, make data-driven decisions to optimize your pricing and resource allocation, and ultimately ensure the long-term sustainability and profitability of your SaaS offering.
How to Leverage Moesif for AI Cost Analysis
Cost analysis is deeply routed in metrics. Whether your AI platform is used through a UI or API, you’ll need an API analytics platform that can be a powerful ally in your quest for efficient AI cost management. While traditionally used for API monitoring and analytics, its capabilities extend to tracking AI usage and associated costs, especially when your AI models are exposed through APIs. Here’s how Moesif can assist you:
-
Granular Usage Tracking: Moesif allows you to track API calls made to your AI models at a granular level. You can see which models are used most frequently, by whom, and how much data they’re processing. This data is invaluable for understanding usage patterns and identifying cost drivers.
-
Cost Allocation: Moesif enables you to associate specific costs with individual API calls. This means you can accurately attribute infrastructure costs (e.g., cloud computing) to the specific models and users responsible for them. This level of cost allocation is crucial for understanding the true financial impact of each AI model.
-
Real-Time Monitoring: With Moesif’s real-time monitoring, you can track AI usage and costs as they occur. This allows you to detect anomalies, such as sudden spikes in usage or unexpected cost increases, and take corrective action promptly.
-
Customizable Dashboards: Moesif provides customizable dashboards that allow you to visualize AI usage and cost data in a meaningful way for your organization. You can create dashboards that track specific metrics, compare costs across different models, and identify trends over time.
-
API Monetization: If you’re offering your AI models as a service, Moesif can help you monetize them through usage-based billing. You can define pricing tiers based on usage metrics like the number of API calls or the amount of data processed. Moesif can then automate the billing process, ensuring you capture the total value of your AI investments.
-
Anomaly Detection: Moesif can be configured to alert you to unusual patterns in AI usage or costs. This proactive approach helps you identify potential issues before they escalate into major problems, saving time and money.
Now, let’s take a closer look at how we can use Moesif to calculate the cost of our AI APIs by looking at cost per token, API call, and user.
Tracking Cost Per Token
One common way to break down AI costs, especially when discussing LLMs, is per-token. This is a standard unit of measurement on platforms such as OpenAI and others. If your AI platform is leveraging one of these third-party platforms under the hood, the cost per token is easy to calculate since it will be shown in your subscription or in the pricing of the model you use. Cost per token becomes more complicated once you try to calculate this within a custom AI engine. While it may require a bit more effort and calculation than using a third-party AI provider, it’s possible to determine the cost per token for your own AI platform. Here’s more particulars on exactly how to calculate this for your platform:
1. Track Total Costs
First, you’ll need to calculate how much your AI API costs to build and support. Here are the areas to consider when calculating this:
-
Infrastructure: Sum up all your monthly infrastructure costs, including servers, GPUs, cloud services, storage, and networking.
-
Software: Calculate your monthly software expenses, including licenses for deep learning frameworks, development tools, and data management tools.
-
Personnel: Total your monthly personnel costs, including salaries, benefits, and training expenses.
-
Data: Estimate your monthly data costs, including acquisition, cleaning, labeling, and maintenance.
-
Research and Development: Factor in any monthly R&D costs related to your AI platform.
2. Track Total Token Usage
Next, we can use Moesif to track how many input, output, and total tokens have been processed within a specific time period. For this calculation, if applicable (some systems may only have a field for total tokens, etc), we will need the following data points:
-
Input Tokens: Sum the total number of input tokens processed by your AI models over a period (e.g., a month).
-
Output Tokens: Sum the total number of output tokens generated by your models over the same period.
To find this data in Moesif, you can create a Time Series report. To do this, click the + New button in the top-left corner of Moesif and select Time Series from the modal that appears. On the Time Series screen, Let’s set the following filter and metrics:
Depending on your API response, this value may be in your body or header field. In this example, we use a value from the headers for X-Input-Token-Count and X-Output-Token-Count.
In the above filter, we look at all API events. For each event, we plot the input tokens (with the value in the X-Input-Token-Count header), the output tokens (from the value in the X-Output-Token-Count header), and the sum of these two amounts to show the total tokens used.
In the output of this Filter and Metrics, you can see the total amount of tokens used in the chart. In the output below, you’ll be able to see the input, output and total tokens used.
In our example, the total number of tokens for the first period we are looking at, July, is 21,787 tokens. We can now take this amount and move to the next step.
3. Calculate Cost Per Token
Now we have all of the metrics and data we need, we can calculate our cost per token. Continuing with our example, let’s say the total monthly costs for your platform are $1,000 (the sum of the costs found in step 1 above), and your models processed 21,787 tokens (input and output combined) in that month (calculated in step 2). Your cost-per-token calculation would look like this:
$1,000 / 21,787 tokens = $0.04589 per token
This will give us our average cost per token, which is $0.04589 per token. If our pricing structure is based on a “per token” price, we can now easily calculate our profit margins at a per-token level.
Tracking Cost Per API Call
Another way we can look at AI costs is the average cost per API call. As explained above, although less common, specific AI platforms may use this pricing. From the user’s perspective, this may look like “$X per [ACTION]”, for example, “$2 per social post generated”, instead of being explicitly considered an API call externally. However, internally, this functionality is delivered via API call, so pricing per API call may make sense. Here’s precisely how to calculate this:
1. Track Total Costs
Like what we discussed in the previous section, you’ll need to calculate how much your AI API costs to build and support. Here are the areas to consider when calculating this:
-
Infrastructure: Sum up all your monthly infrastructure costs, including servers, GPUs, cloud services, storage, and networking.
-
Software: Calculate your monthly software expenses, including licenses for deep learning frameworks, development tools, and data management tools.
-
Personnel: Total your monthly personnel costs, including salaries, benefits, and training expenses.
-
Data: Estimate your monthly data costs, including acquisition, cleaning, labeling, and maintenance.
-
Research and Development: Factor in any monthly R&D costs related to your AI platform.
2. Track API Usage by API Call
Next, we can use Moesif to track how many API calls have been processed within a specific time period. To find this data in Moesif, you can create a Time Series report. To do this, click the + New button in the top-left corner of Moesif and select Time Series from the modal that appears. On the Time Series screen, Let’s set the following filter and metrics:
In the above filter, we look at all API events. In the resulting chart, we will see all of the API calls per month displayed. In this specific example, we are looking at all API calls but you can also refine this by endpoint as well to get a more precise cost.
In the output of this Filter and Metrics, you can see the total amount of API calls that occurred in the previous month (July) in the chart.
In our example, the total amount of API calls in the first period we are looking at, which is July, is 9,956 tokens. We can now take this amount and move to the next step.
3. Calculate Cost Per API Call
Now we have all of the metrics and data we need, we can calculate our cost per API call. Continuing with our previous example, let’s say the total monthly costs for your platform are $1,000 (the sum of the costs found in step 1 above), and your APIs processed 9.956 calls in that month (calculated in step 2). Your cost per API call calculation would look like this:
$1,000 / 9,956 tokens = $0.1004
This will give us our average cost per API call, which is $0.1004 per call. If our pricing structure is based on a “per API call” price, we can now easily calculate our profit margins at a per-API call level.
Tracking Cost Per User
Lastly, for instances where you are charging so much per seat, you may be interested in figuring out how much your AI costs per user. For instance, if you charge $15.99 per seat for all-you-can-eat access on your platform, you’ll want to know the average cost per user to ensure you meet your expected profit margins. For this, we will need to understand our user count and spread our cost over the number of users on the platform. Here’s precisely how to do this:
1. Track Total Costs
Like what we discussed in the previous sections, you’ll need to calculate how much your AI API costs to build and support. Here are the areas to consider when calculating this:
-
Infrastructure: Sum up all your monthly infrastructure costs, including servers, GPUs, cloud services, storage, and networking.
-
Software: Calculate your monthly software expenses, including licenses for deep learning frameworks, development tools, and data management tools.
-
Personnel: Total your monthly personnel costs, including salaries, benefits, and training expenses.
-
Data: Estimate your monthly data costs, including acquisition, cleaning, labeling, and maintenance.
-
Research and Development: Factor in any monthly R&D costs related to your AI platform.
2. Track API Usage by Unique Users
Next, we can use Moesif to track how many users are active on the platform within a specific time period. To find this data in Moesif, you can create a Time Series report. To do this, click the + New button in the top-left corner of Moesif and select Time Series from the modal that appears. On the Time Series screen, Let’s set the following filter and metrics:
In the above filter, we look at all API call events that have come into Moesif. From here, we further refine this by selecting Unique Users as our value selected from the Metrics dropdown. This will let us know how many users used our platform.
In the output of this Filter and Metrics, you can see the number of unique users that have accessed the platform per month. In the output below, you’ll see the exact amount of users that we can use to calculate the AI cost per user.
In our example, the total number of unique users in the first period we are looking at, which is July, is 601 users. We can now take this amount and move to the next step.
3. Calculate Cost Per User
Now we have all of the metrics and data we need, we can calculate our cost per user. Continuing with our example, let’s say the total monthly costs for your platform are $1,000 (the sum of the costs found in step 1 above), and we have had 601 unique users on the platform in that month (calculated in step 2). Your cost per user calculation would look like this:
$1,000 / 601 users = $1.6638 per user
This will give us our average cost per user, which is $1.6638 per user. If our pricing structure is based on a “per user/seat” price, we can now easily calculate our profit margins at a per-user level.
Notes for Further Refinement
Although the above examples are great and will get you a good idea of your average costs, there are a few ways to dial things in a bit more and a few factors to consider.
First, you may want to narrow down your cost analysis to specific endpoints since the underlying AI may cost more to run on one API versus another. To do this, you would simply change any of the use cases outlined above and scope the analysis to specific endpoints. This would include everything from calculating the total costs to making sure that your filter in Moesif is set to only look at data for a specific endpoint. This would be done in the Filter for the Time Series, specifying “URI Route is /X” with “X” being the API endpoints you want to scope your results to.
Secondly, sometimes licenses or seats may be granted at the company level so it may make sense to look at the Unique Companies versus Unique Users metric that we used in our per-user calculation. This will allow you to calculate your cost at a higher level if it is useful to your pricing strategy.
Lastly, we looked at average costs, which tends to be more effective and smooths out cost calculations a bit more. However, there may be some reason for being more specific and calculating costs at an exact amount for each AI interaction. This can be somewhat challenging, especially for high-volume services. If your AI platform spins up infrastructure per client, then this would likely be possible and may be advantageous, however, with shared infrastructure average cost is the most effective way to do a cost analysis for AI platforms.
Conclusion
In the era of AI-powered innovation, understanding and managing costs is not just a financial necessity; it’s a strategic advantage. AI cost analysis empowers you to make informed decisions, optimize your resources, and ensure that your AI initiatives are not only cutting-edge but also financially sustainable.
By identifying cost drivers, tracking usage patterns, and optimizing resource allocation, you can unlock the full potential of AI while keeping your budget in check. Remember, the journey of AI cost analysis is ongoing. As your AI projects evolve and scale, so too will your costs. Continuous monitoring and optimization are key to long-term success.
If you’re looking for a powerful tool to streamline your AI cost analysis, Moesif is a great platform for collecting and analyzing data around various aspects of your customer’s AI usage. With its granular tracking, real-time monitoring, and customizable dashboards, Moesif provides invaluable insights into your AI spend and revenue. Whether you’re a startup experimenting with AI or an enterprise deploying complex AI systems, Moesif can help you gain control of your AI costs and maximize the return on your AI investments.
Ready to take the next step in understanding your AI costs? Sign up for a free trial of Moesif today and discover how easy it is to understand and optimize your AI costs.