Cultivate Success: The Ultimate Guide to Growing AI Apps
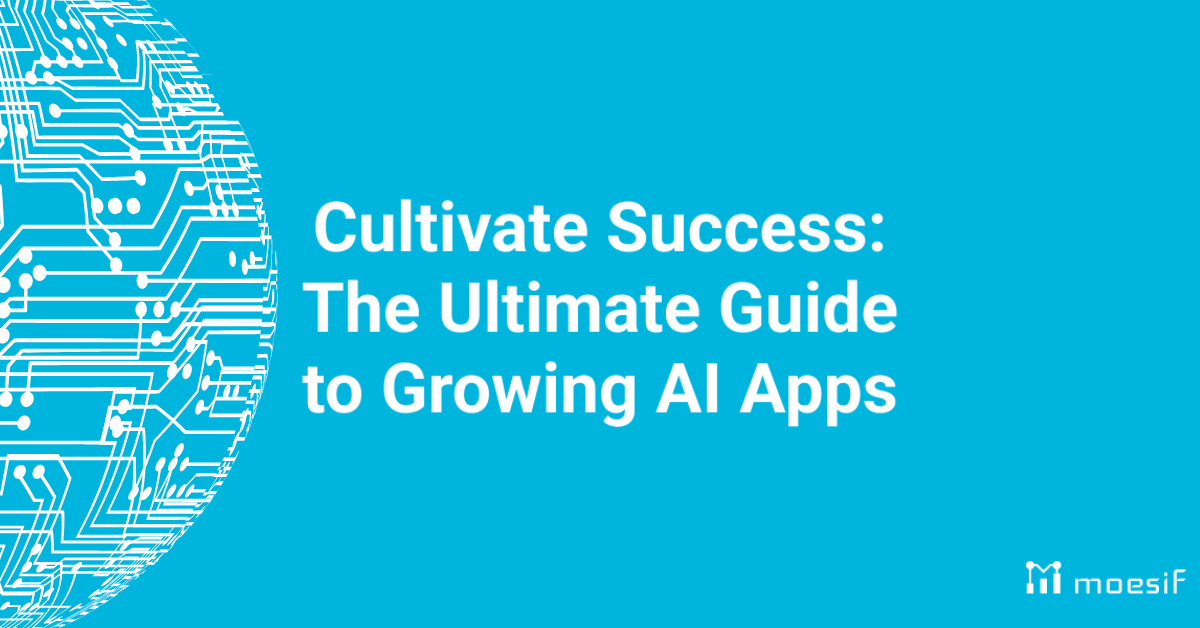
Commercializing an AI-based product requires turning technology into a marketable product, navigating challenges from development to market entry. Appealing to enterprise buyers is crucial for sustainable, continuous growth, as their interest not only validates your product’s value but also lays the foundation for long-term success and scalability. More specifically, the larger the businesses of your potential customers are, the more of a monopoly your product likely has in the market. By strategically aligning your product offering with enterprise buyers’ needs, you can ensure a robust market presence and pave the way for impactful industry adoption of your AI solutions. How to make money from AI products has everything to with how AI companies or AI startups position their generative AI tools to the greater public.
When looking at the initial investment and cost of building an AI solution, it’s important to assess not only the financial feasibility but also the strategic allocation of resources and costs associated with AI model maintenance. By considering factors such as data acquisition, algorithm development, machine learning infrastructure, and potential unknown challenges in the AI landscape, you can better position your product as a viable, long-term solution to your user’s problems.
When it comes to the cost of launching and maintaining an artificial intelligence model or product, it becomes evident that technological infrastructure and robust research can significantly contribute to the overall development and investment required to successfully develop and launch an AI tool.
Enterprise customers generally require a “tried and true” solution, one that instills confidence rather than doubt. Because the costs of enterprise contracts for AI software can be extremely high due to resource investment (on both ends of the deal), a viable proof of concept (PoC) and trial can be the difference between a signed deal and no customer. This necessitates a comprehensive understanding of how each element of your big data infrastructure impacts the project’s financial scope as well as what costs can be eaten by your organization versus which can be monetized and offset by your enterprise users.
Emphasizing the importance of a realistic budget and cost-effective strategies is critical for long-term success, and longevity is paramount for ensuring enterprise interest. Building an AI system can be expensive; the complexity of AI algorithms and models often requires significant computational power, which can demand high-performance hardware, leading to substantial upfront costs. Beyond this, hiring knowledgeable people to fill data scientist or data analyst roles can be costly.
Additionally, the need for continuous, uninterrupted data storage, processing, and model training, coupled with rapid advancements in AI technology all require ongoing financial and technological investments in both hardware and software components (and talent!). These financial constraints can be prohibitively expensive for newer organizations to set up, let alone maintain their newfound business idea. Taking part in the AI revolution may seem like a natural step for your organization, but the associated costs of maintaining AI companies can be prohibitive.
Taking an AI technology live involves distinct challenges. Initially, ensuring the readiness of the product and any associated AI model(s) is the most crucial step, encompassing rigorous testing, addressing potential bugs, and optimizing performance to deliver a seamless and accurate user experience that will make even the most critical users content. Once a product is viable, onboarding users effectively becomes equally important, requiring intuitive user design, comprehensive but accessible documentation, and responsive customer service. Striking the right balance between accessibility and security while adhering to compliance standards can be the difference between a successful product with data security and a lawsuit in waiting.
Tailoring your marketing and sales strategies to enterprise buyers is also a large part of successful adoption of any natural language processing product. For enterprise clients, the emphasis should be on scalability, security, customization, and alignment with their specific use cases. Effective marketing involves showcasing the AI powered tool’s ability to solve complex challenges, increase efficiency, and provide a significant return on investment, likely through a PoC or sandbox trial. Leveraging Moesif’s user insights during this phase adds a valuable dimension to the deal by offering real-time monitoring on user behavior and API usage, providing your business with actionable data for PoC development and refining strategies for optimal targeting and new market penetration.
Moesif’s deep API analytics delve into the nuances of customer behavior and usage patterns, making it easier for you to detect subtle changes and triggers that may signal potential churn or blockages for your AI application. Proactive alerts serve as a preemptive tool for businesses to take timely action and retain valuable customers through data-driven workflows. Moesif enables non-technical business teams to intervene promptly when addressing diminishing customer value, offering insights that facilitate strategic decision-making to enhance customer satisfaction and prolong brand loyalty.